What Is a Data Fabric: Definition, Architecture, and Benefits
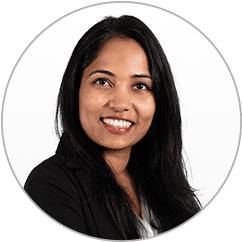
Table of contents
With so many terms out there such as data fabric, data lakes, data lakehouses, data mesh, and cloud enterprise warehouses, it can be overwhelming for organizations to understand and decide what works for their current architecture and what is best suited for their data management and data governance strategy. To start off, we would like to focus on a recent methodology such as data fabric, that can be included in your data management strategy.
At STAEDEAN, we build solutions for end-to-end data management, data integration, data governance, EDI integration, and data analytics that have been embedded in Microsoft Dynamics 365 ERP. So, we understand that with all these terminologies, and updates in the way organizations store, organize, manage, and control their data, it is important for our customers and prospects to understand terms such as data fabric to succeed in becoming a data-driven organization.
Therefore, in this blog, we will explain:
- Why do organizations need data fabric?
- What is a data fabric?
- Data fabric architecture and components
- Data fabric benefits
- Is data fabric the right way forward?
Why do organizations need data fabric?
As data has been exploding across organizations around the world and multiple tools exist to help organizations store structured and unstructured data in storage systems that we mentioned earlier, data management and governance have become an arduous task to manage. Organizations have to often deal with challenges in managing data in disparate systems, security risks, and organizing and cleaning data as a result.
A data fabric is the answer to these challenges. In the Essential Guide to Data Fabric, Gartner estimates that by 2024, “data fabric deployments will quadruple efficiency in data utilization while cutting human-driven data management tasks in half”.
So, what is a data fabric?
The data fabric is an architectural approach or a methodology that allows you to govern all sources of data whether it is on-premises systems, multiple cloud environments, or SaaS applications, by adding a virtualization layer without moving or copying data. Data integration strategy and solutions play a key role in building a robust data fabric.
Gartner defines data fabric as “a design concept that serves as an integrated layer (fabric) of data and connecting processes. A data fabric utilizes continuous analytics over existing, discoverable, and inferenced metadata assets to support the design, deployment, and utilization of integrated and reusable data across all environments, including hybrid and multi-cloud platforms.”
Using data fabric for data management allows you to access data across systems and also copy or move data when needed using a strategy and tools. It establishes a means to identify and synchronize data from multiple systems and applications to determine connections between the available data. Data fabric focuses on the synchronization of information across data pipelines by using active metadata by implementing processes that make data sharing and access easier and avoids data silos and data duplication across systems. This approach makes data access easier, efficient, and also significantly improves decision-making.
Data fabric architecture and components
As data creation grows year on year across organizations with an increase in the number of business systems, on-premises and cloud databases, and applications, all hosting data, the need to have an architecture to manage data across systems becomes more imperative for organizations.
A data fabric creates a virtualization layer by using APIs and integration tools to ensure data access and availability are possible across the organization. It pulls data from multiple systems, databases, and apps and focuses on data movement, transformation, and synchronization.
There are seven layers in the data fabric including:
1. Data management layer
Every business system, whether it is your Enterprise Resource Planning software or Customer Relationship Management/Customer Experience software, has a database that could be hosted on Azure, AWS, or another database. Additionally, you will have structured and unstructured data in multiple cloud and/or on-premises environments. In this layer, the important thing is to first identify data sources for ingestion and then have processes for deduplication, compression, migration, and tiering.
2. Data security layer
While data integration makes data accessible, there needs to be data protection practices and rules in place to ensure security. There needs to be a way to secure and back up all data. In the case of older information, there need to be methods to archive data. And when there is a breach or a data loss, then data recovery practices need to be carried out.
3. Data access layer
This layer makes data available based on the security rules and processes in place in the previous layer. Using APIs and an integration solution, your teams can access data across diverse data sources from the cloud to data lakes. This layer makes it possible for querying, updating, deleting, sharing, or moving data.
4. Data transformation layer
As data that is ingested in the first layer could be in a structured, unstructured format or somewhere in the middle, to make sense of that data, data transformation is important. Implementing data processes that can transform, sort, and clean that data into a coherent format is what comes next. This will help people to then establish relationships between the various data sources in an organization.
5. Data visualization layer
Before data can be analyzed, data needs to be cleaned and monitored with data governance practices in place. Advanced technologies such as Artificial Intelligence and Machine Learning (AI & ML) to build dashboards can be set up for data monitoring and visualization. This will help you with data preparation and visualization which will make it easier to derive valuable business insights from your extracted data.
6. Data transport layer
This layer establishes practices for data movement across the fabric to ensure no duplication, no disruptions in business, and compression wherever needed. These practices ensure that the data fabric is in motion without any issues or security risks.
7. Hosting the data fabric and monitoring your integrations
Although this is external to the data fabric architecture, it is an important component. The data fabric can be hosted on the cloud or on-premises, as long as data integration is possible between your architectural landscape and business systems and information is easily synchronized without any data silos. Data fabric can also be hosted in a hybrid cloud or multi-cloud environment. Besides hosting the data fabric, monitoring your integrations is also an important element of strengthening your data fabric after it has been orchestrated.
Data Fabric Benefits
Based on the Data Fabric Market Outlook by Future Market Insights, the global data fabric market size is estimated to be worth US$ 2.43 billion in 2023 and is forecasted to grow at a CAGR of 15.54% for the next 10 years.
If your organization does decide to be part of this growth, then you will witness the benefits of implementing a data fabric architecture as listed below.
Lesser data silos and improved access
Data silos result in inefficiencies and a lack of visibility of the bigger picture. As the core of the data fabric is to connect all data sources and make data accessible, identifying and reducing data silos is the most important step in the process of establishing a data fabric architecture. A data fabric allows you to share data regardless of location, system, based on data governance rules.
Improved data governance
While establishing data governance is important, implementing it using a data fabric architecture can help your organization automate the process.
Holistic view of data
While integrating certain systems to improve efficiency might be a business need but connecting your entire application landscape can give you a holistic view of your data and a data fabric allows you to do that.
Improved compliance
As part of data governance, adhering to security protocols while data access, sharing, migration, deletion, and archiving have to be taken into account while establishing a data fabric.
Whether you want to centrally manage your master data, or apply data rules to improve data security, add access protocols, a data fabric can be built keeping these in mind to ensure data compliance based on industry or geographical regulations.
Improved data analytics
Since it allows you to connect structured and unstructured data, mine what is valuable, and visualize it, it can considerably help you improve analytics and business intelligence.
Is data fabric the right way forward for your organization?
We hope we have helped you understand the meaning, core components, and benefits of investing time and money building a data fabric architecture. As a next step, it might help to learn more about data governance best practices, and a data governance framework before exploring a data fabric architecture for your organization.
If you would like to learn all about data governance in detail, please download our complete guide to data governance from the link given below.