What Is a Data Lifecycle? What Are the Stages in a Data Lifecycle?
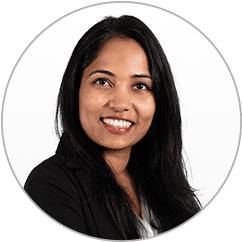
Table of contents
Artificial Intelligence or AI has been the biggest technology trend in 2023 making leaps with the introduction of a multitude of AI platforms that can do a variety of tasks from creating vivid portraits to writing code. Organizations that want to ride the AI wave need to first assess their data lifecycle and perfect the processes at each stage. Without the right processes for data management, investing in AI would be futile.
Identifying and understanding your data lifecycle will propel you in the direction of becoming AI-ready. You can then identify the challenges in each of those stages and look at strategies to overcome them.
At STAEDEAN, we understand the challenges in the data lifecycle as we have developed Data Management Solutions for Microsoft Dynamics 365 Finance & Supply Chain Management. Our no-code embedded solutions range from EDI to data analytics. Our solutions solve challenges in various stages such as missing data during creation, insecure access after data has been created, difficulty in distributing transactional master data across companies, managing data quality across the data lifecycle, and deriving business insights from that data.
Data is created, edited, accessed, distributed, and stored every single day. But when you do not understand a data lifecycle it is difficult to resolve challenges at each stage. Therefore, to help our readers, we will explain what a data lifecycle is and the several stages in this article.
What is the data lifecycle?
Data is present in various business systems. Now imagine your data’s life as a story, it is born when you create it, grows when you update or edit it, is traveling when you distribute it, and eventually ends when you delete it. That's the data lifecycle in very simple terms.
In business, the data lifecycle is the journey of information from its creation to its deletion. It starts with creation, then moves to maintenance, sorting, processing, access, distribution, updating, storing, visualization, analytics, and finally archiving and deletion. Since businesses have to manage each of these stages every day, it is important to manage data efficiently throughout these stages.
Different organizations may have different stages and strategies to ensure data governance across the data lifecycle. There might be different teams involved at each of these stages, with limited data access for crucial data. Regional data might be updated or distributed locally to ensure data accuracy.
Organizations and standards bodies like ISO (International Organization for Standardization) and NIST (National Institute of Standards and Technology) provide frameworks and guidelines for managing the data lifecycle to ensure security and regulated access. An example of this would be the GDPR regulation in Europe that ensures organizations follow regulations for data protection and privacy.
Now that we understand what a data lifecycle is, let’s dive into each stage in the section below.
What are the stages in the data lifecycle?
1. Data Generation
This is where data is imported or created in a business system. It could be through various means such as but not limited to, incoming electronic data interchange (EDI) messages, manually created records in the ERP, imported Excel files, sensors, transactions, customer forms on your website, etc.
2. Data capturing and maintenance
This stage is important to ensure data accuracy, completeness, and validity because the quality of data is dependent on the rules applied, and the verification process. The processes for data capture and maintenance over time impact data accuracy and consistency too. Collaboration at this stage among team members to ensure data completion and validation helps improve data quality.
3. Data processing
Raw data often needs to be transformed and processed to extract valuable insights. This can involve cleaning, aggregating, or enriching the data.
4. Data sorting and access
Ensuring data access in accordance with the data governance strategy for security and compliance is important to limit data access to ensure critical data is safeguarded. Data classification makes it easier to publish, store, visualize, and analyze that data.
5. Data distribution and publishing
After data has been cleaned, validated, and sorted based on the organization’s data governance strategy, it can be distributed using a centralized or decentralized master data management strategy or published to ensure employees and stakeholders have access to master data.
6. Data updates
After some time data might need to be updated to ensure accuracy or completeness. Managing these updates in a secure manner with processes and solutions in place ensures fewer data breaches, fraud, and theft. Therefore, it is important to have an outlined process for this stage.
7. Data visualization reporting, and analytics
This is the stage where value is derived from cleaned data. You can leverage technologies such as Artificial Intelligence and Machine Learning to transform complex data into visual reports that can help you analyze your data and chart out reports. Organizations could use analytics tools or statistical methods based on their preference. This will help them derive powerful business insights through reports, graphs, dashboards, or other means that make it easier to visualize data. These insights are often shared using secure mechanisms across departments or with key stakeholders involved. Based on the analysis, organizations can make impactful decisions that can fuel growth and help them scale their business.
8. Data storage, archiving or deletion
Data needs a secure home and can be stored in data warehouses, databases, or similar storage systems. This stage involves deciding whether the data needs to be stored, archived, or deleted and the period for the same. Older data that might not be used but is required for reference can be archived for historical purposes. Data that is outdated or reached the end of its lifecycle needs to be securely deleted in keeping with data protection regulations.
How will the data lifecycle differ for organizations?
The fundamental stages of the data lifecycle are quite similar across business systems and organizations. However, the specific details and emphasis on each stage may vary depending on the nature of the business, industry regulations, and the organization's goals and processes.
Industry Differences
Industries with strict regulatory requirements, such as finance or healthcare, will need to focus on data security and compliance.
Industries driven by innovation such as technology and manufacturing, may prioritize data analytics and business intelligence.
Organizational Size
Larger organizations often deal with complex data ecosystems and might want to migrate from legacy systems to more advanced cloud-based systems or maybe focus on establishing data governance processes. Smaller organizations on the other hand might have a simpler data lifecycle but could face different challenges such as integrating systems and applications.
Data-Centric vs. Process-Centric Organizations
Data-driven organizations have data at the core of their operations and focus on all aspects of data management and analytics to derive high value from their data. Process-centric organizations are still trying to make that leap but struggle as data is secondary to managing business processes and operations.
Infrastructure
Organizations that have migrated to more modern cloud-based systems will have different priorities in managing their data lifecycle versus organizations that are still using legacy on-premises systems.
Your organization may feature in more than 1 of the above-listed categories. But despite these variations, the core stages including data creation, processing, access, publishing, storage, and deletion of data are fundamental for every organization. You can tailor and prioritize these stages depending on your organization’s business requirements and objectives.
Are you looking to resolve challenges in your data lifecycle?
We have shared 8 stages in the data lifecycle in this article. It is possible organizations might not need all of these stages. While the fundamental stages of the data lifecycle are quite similar across organizations, the way you manage each of the stages, the solutions you use, and such details will vary depending on the nature of your business, the industry regulations that you need to follow, and your organization's goals.
However, any organization can strive to improve its data lifecycle management by using feedback from your teams managing each of these cycles at regular intervals. This will help you make improvements at each of the stages in your data lifecycle. Once you are able to streamline your data lifecycle management and you have clean data to derive value from, your organization can consider investments in AI and automation.
If you are interested in reading more about the data lifecycle, download our whitepaper from the link below. Alternatively, if you are interested in knowing more about our Data Management Solutions for Microsoft Dynamics 365 Finance and Supply Chain Management, browse our solution pages.